Industrial scheduling has historically depended on human expert knowledge or problem-specific dedicated systems. Although the problem definition is deceptively simple, it is an NP-hard problem as the quantity of possible states on a planning board rises in proportion to the number of orders to the power of number of tasks.
A generic model for the problem involves a set of orders that require processing time on one or more resources with specific constraints.
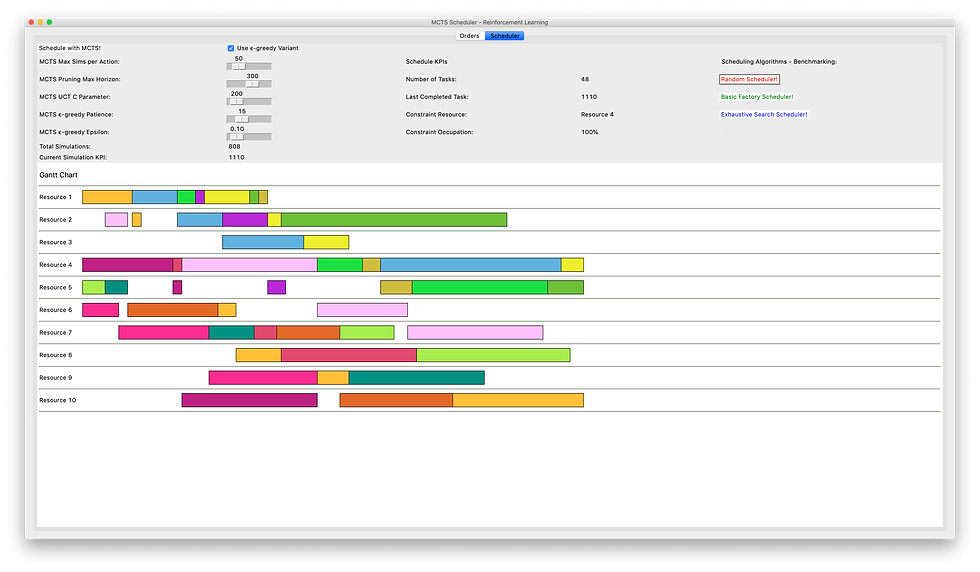
Monte Carlo Tree search can be applied to find near-optimal solutions to the scheduling problem, as preparation for a deep learning function similar to the technique applied to Alpha-Go (deep reinforcement learning). I discuss this approach in detail here.
Comments